About
Who are we?
The Cancer Control PopSim (Population Simulation for Healthcare Decisions) team is comprised of academic public health researchers who are focused on mathematically modeling the expected impact of health-related evidence-based interventions (EBI) and health policy changes on population health outcomes, particularly within the context of colorectal cancer (CRC) screening and outcomes. We represent the Modeling Evidence-Based Intervention (EBI) Impact Workgroup within the Cancer Prevention and Control Research Network (CPCRN), a national thematic research network dedicated to reducing the burden of cancer and addressing health disparities within cancer care. This network is part of the Prevention Research Centers (PRC) program funded by the Centers for Disease Control and Prevention (CDC). We work closely with, and receive support from, the Division of Cancer Prevention and Control (DCPC) within the CDC.
Together, our team members contribute expertise related to cancer prevention and outcomes, health disparities, simulation modeling, health systems engineering, economic evaluation, health policy, and the design and implementation of EBIs. Current contributors are affiliated with the University of North Carolina at Chapel Hill, North Carolina State University, Oregon Health & Science University, University of Florida, and the CDC.
What do our population healthcare models do?
We use population-level models that create a real-world simulated cohort of individuals living in a particular state, with features like age, sex, race/ethnicity, and insurance status that resemble the Census for that state. These models are capable of predicting the short- and long-term health outcomes (for example, likelihood of developing colorectal cancer) and associated costs of current standards of care (for example, the expected cost of treating colorectal cancer), and how these outcomes are expected to change over time as a result of specific healthcare interventions and policies. This approach allows for estimating and comparing the differential costs and health impacts of strategies intended to improve population health outcomes.
Our models are currently designed to simulate colorectal cancer-related outcomes within the state populations in North Carolina and Oregon, as well as subgroups within these states (for example, African Americans or Medicaid-insured people). We can estimate the impact of implementing evidence-based interventions (for example, mailing a stool kit to age-eligible individuals who are overdue for colorectal cancer screening) or health policy changes (e.g. insurance expansion) on cancer screening, cancer occurrence, cancer death, and related costs for these populations. Right now, we focus on estimating how specific interventions and policies of interest to decision makers will affect the percentage of age-eligible individuals up-to-date with colorectal cancer screening, the number of cancer cases prevented, and total costs of cancer screening, cancer diagnosis, and cancer treatment, compared to current standards of care (i.e., status quo).
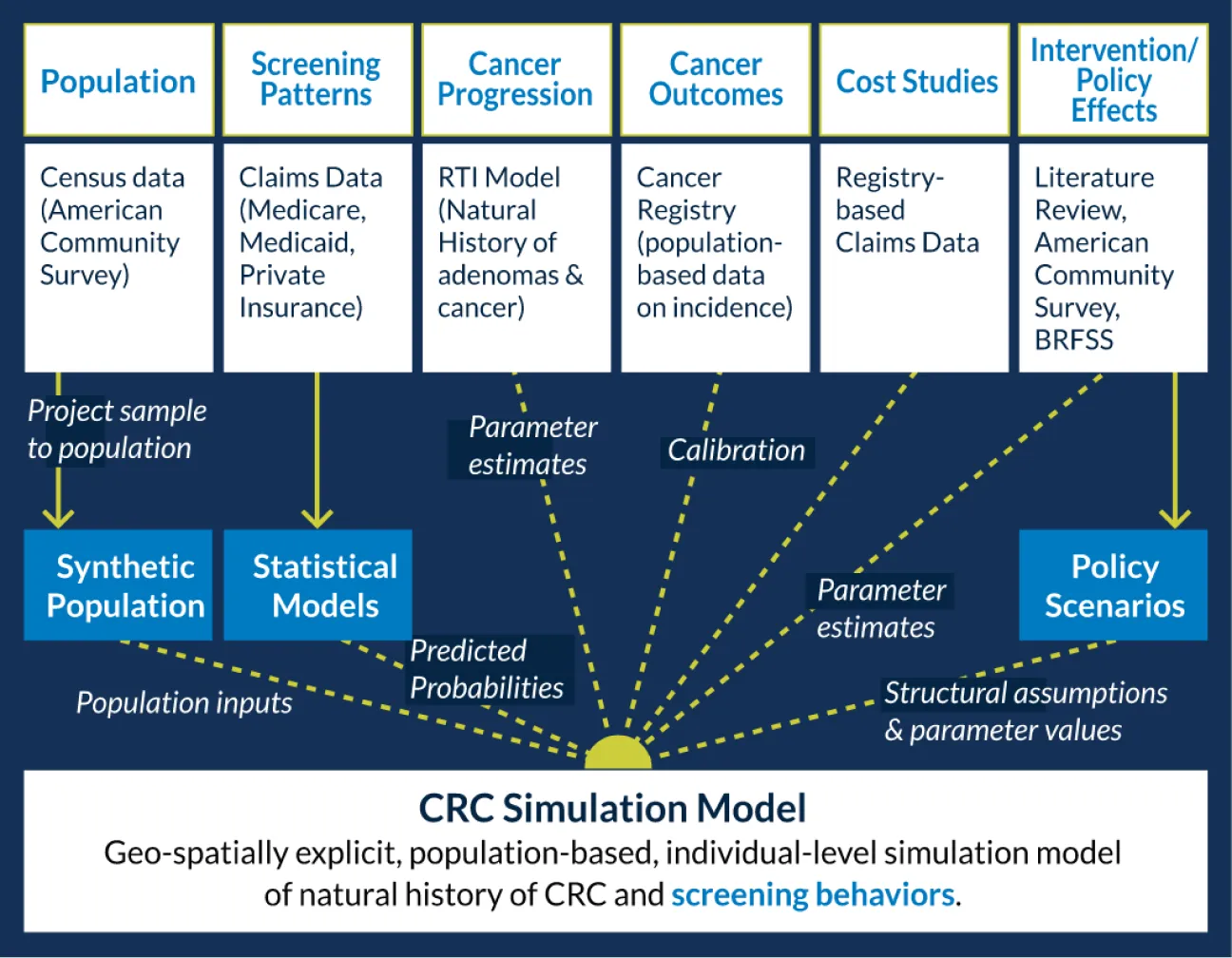
Why are these models needed?
Our PopSim models, like some other mathematical simulations in healthcare, are important for understanding which public health strategies are expected to have the greatest value in terms of health outcomes and cost. In addition, our models provide insight into how these findings may vary by location or subgroup. For example, is insurance expansion expected to differentially benefit people who have historically been underserved, such as people of color or rural-dwelling people, in terms of cancer-related outcomes?
Our PopSim models are intended to be used as a “virtual world” in which to simulate population-level health and cost outcomes associated with alternate assumptions about population demographics, disease determinants, effects of clinical interventions or policies on health, and costs of healthcare use over time. This information is needed to provide state-level, system-level, and community-level data to decision-makers who are in positions to invest in public health interventions and policies to reduce cancer-related morbidity, mortality, and disparities. In particular, decision-makers can use this information to determine which strategies are most optimal and value-added depending on the target region and/or subgroup of interest.
Learn more about the CRC modeling approach.
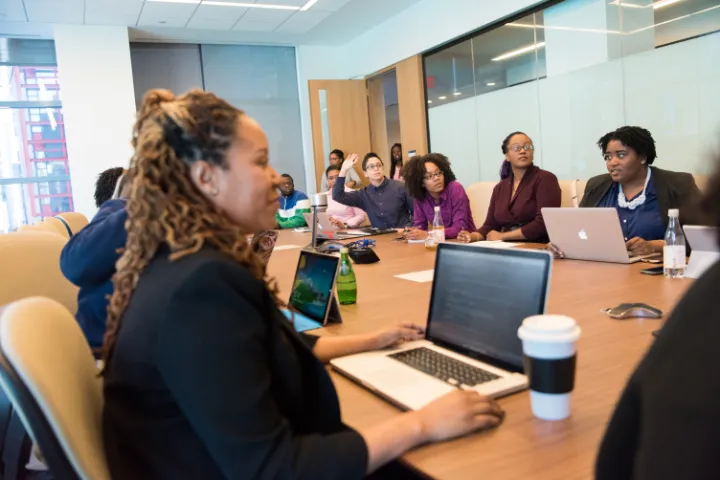
Affiliated entities
- Cancer Prevention and Control Research Network
- UNC Gillings School of Public Health: Health Policy and Management
- UNC Center for Health Promotion and Disease Prevention
- UNC Lineberger Comprehensive Cancer Center
- Prevention Research Centers
- CDC
- University of Florida
- NC State University: Edward P. Fitts Department of Industrial and Systems Engineering
- OHSU: Knight Cancer Institute
- OHSU: Oregon Rural Practice-Based Research Network